Mapping Typical Urban LULC from Landsat Imagery without Training Samples or Self-Defined Parameters
Li, H.; Wang, C.; Zhong, C.*; Zhang, Z.; Liu, Q. Mapping Typical Urban LULC from Landsat Imagery without Training Samples or Self-Defined Parameters. Remote Sens. 2017, 9, 700.Doi:10.3390/rs9070700
Abstract:
Land use/land cover (LULC) change is one of the most important indicators in understanding the interactions between humans and the environment. Traditionally, when LULC maps are produced yearly, most existing remote-sensing methods have to collect ground reference data annually, as the classifiers have to be trained individually in each corresponding year. This study presented a novel strategy to map LULC classes without training samples or assigning parameters. First of all, several novel indices were carefully selected from the index pool, which were able to highlight certain LULC very well. Following this, a common unsupervised classifier was employed to extract the LULC from the associated index image without assigning thresholds. Finally, a supervised classification was implemented with samples automatically collected from the unsupervised classification outputs. Results illustrated that the proposed method could achieve satisfactory performance, reaching similar accuracies to traditional approaches. Findings of this study demonstrate that the proposed strategy is a simple and effective alternative to mapping urban LULC. With the proposed strategy, the budget and time required for remote-sensing data processing could be reduced dramatically.
Keywords: Urbanization; remote-sensingindices; unsupervised classification; automated supervised classification.
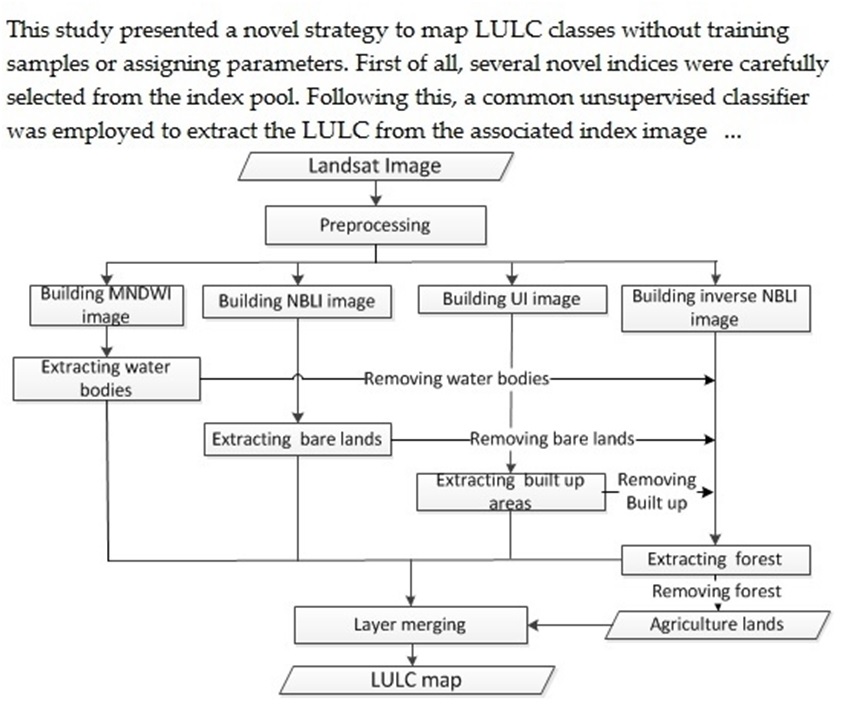